D. Post Processing and Cross Validation
1. Modeling and Quantification: Part 3
Moderators: Adriaan A. Lammertsma and Hidehiro Iida
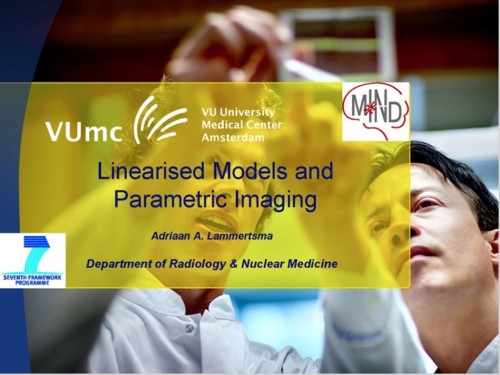
Please click the slide image to open and view the presentation. Please note- the slides in the presentation can be advanced using the navigation controls on the presentation frame.
Presentation 1: Linearised Models and Parametric Imaging
Adriaan A. Lammertsma
Radiology & Nuclear Medicine, VU University Medical Center, Amsterdam, Netherlands
Learning Objectives:
- Understand the advantages of linearised models
- Understand the limitations of linearised models
- Obtain an overview of the various linearized methods available
- Understand why linearized models are needed for parametric imaging
Molecular in vivo imaging techniques provide non-invasive measurements of regional tissue uptake and clearance of selected molecules. The kinetic behaviour of these molecules (tracers, probes) depends on several underlying physiological and/or biochemical processes. Tracer kinetic modelling is required to extract quantitative information on the specific molecular process under study.
PET provides for accurate measurements of regional tissue concentrations of tracers labelled with a positron emitter. Tracer kinetic models are needed to extract quantitative values of an underlying molecular process from these measurements. In practice, essentially all models are compartment models and physiological or biochemical parameters are obtained by fitting measured time-activity curves to the non-linear operational (model) equation. The most common parameters of interest are volume of distribution (VT) and non-displaceable binding potential (BPND), providing measures of total and specific uptake in tissue, respectively, normalized to activity delivered (input function).
In general, data are fitted using standard non-linear regression algorithms. Non-linear regression, however, is rather slow and sensitive to noise, and therefore it usually is applied only to region of interest data (low noise level). Ideally, fits should be performed at the voxel level, thereby enabling the generation of parametric images (i.e. images of the parameter under study). Although, in theory, it is possible to fit individual voxel data, in practice this is not feasible. Apart from being too slow, non-linear regression would result in very noisy images with (many) outliers. To generate parametric images, linearization of the model equations is required. Several methods have been developed using either graphical or multi-linear regression approaches: Patlak plot- direct integration of model equations and Logan plot- multi-linear regression analysis. As these methods involve some sort of transformation of variables, they are “approximations” of the full compartmental equations and require validation for specific applications.
Another approach is the basis function method, which in theory can be used for all compartment models. The method is especially known for its implementation of the simplified reference tissue method. The basic principle of the basis function method is to pre-calculate any convolution in the model equation for a set of “basis” functions that cover the entire range of physiological values. For each pre-calculated convolution, the model equation becomes linear and its coefficients can be obtained by linear regression. The final parameters are then obtained by selecting the linear fit with the lowest residual sum of squares. The main advantage of parametric methods is that they allow for parameter estimations at the highest possible (scanner) resolution. Nevertheless, as they are linearizations, parametric methods need to be validated against their full compartmental counterparts. For each tracer, several parametric methods should be investigated, as no single method is ideal for all applications.
Relevant Publications:
- Blomqvist G (1984) On the construction of functional maps in positron emission tomography. J Cereb Blood Flow Metab 4:629-632
- Gunn RN, Lammertsma AA, Hume SP et al (1997) Parametric imaging of ligand-receptor binding in PET using a simplified reference region model. NeuroImage 6:279-287
- Ichise M, Toyama H, Innis RB et al (2002) Strategies to improve neuroreceptor parameter estimation by linear regression analysis. J Cereb Blood Flow Metab 22:1271-1281
- Ichise M, Liow JS, Lu JQ et al (2003) Linearized reference tissue parametric imaging methods: application to [11C]DASB positron emission tomography studies of the serotonin transporter in human brain. J Cereb Blood Flow Metab 23:1096-1112
- Logan J, Fowler JS, Volkow ND et al (1990) Graphical analysis of reversible radio-ligand binding from time-activity measurements applied to [N-11C-methyl]-(-)-cocaine PET studies in human subjects. J Cereb Blood Flow Metab 10:740−747
- Logan J, Fowler JS, Volkow ND et al (1996) Distribution volume ratios without blood sampling from graphical analysis of PET data. J Cereb Blood Flow Metab 16:834–840
- Patlak CS, Blasberg RG, Fenstermacher JD (1983) Graphical evaluation of blood-to-brain transfer constants from multiple-time uptake data. J Cereb Blood Flow Metab 3: 1-7
Presentation 2: Applications of Modeling and Quantification in the Brain
Steven Meikle
Brain and Mind Research Institute, University of Sydney, Sydney, NSW, Australia
Learning Objectives:
- Understand the importance of parameter estimation in clinical and research applications of neurological PET
- Understand how to determine the most suitable quantitative method for a given neurological PET application
- Appreciate the challenges and limitations of quantitative parameter estimation techniques in neurological PET, as well as the importance of the information they provide
This presentation explores the role of quantitative kinetic parameter estimation in molecular imaging of the brain, with a particular focus on positron emission tomography (PET). Examples will be drawn from clinical and research applications of PET where quantitative methods are not only useful but, in certain cases, essential to answer the question at hand. These include applications requiring the estimation of regional cerebral blood flow, cerebral rate of oxygen and glucose metabolism, receptor density and receptor occupancy. Case studies will also be chosen to illustrate the broad range of methodological approaches described in the literature, including the linearization methods highlighted in the preceding lecture, and the challenges encountered in using these methods in practice.
Presentation 3: Quantitative Assessment of Regional Myocardial Perfusion using PET
Hidehiro Iida
Department of Investigative Radiology, National Cerebral and Cardiovascular Center, Suita City, Japan
Learning Objectives:
- To learn basic principles and assumptions when assessing regional myocardial blood flow by means of a compartmental modelling approaches.
- To learn practical procedures to assess quantitative regional myocardial blood flow using PET and typical radio tracers such as 15O-water, 13N-ammonia and others.
- To learn limitations and possible error factors in the estimated myocardial flow values.
Regional myocardial blood flow is defined as a perfusion rate over a unit time per unit mass of the myocardial tissue, and can often be a critical factor in various pathophysiological conditions. Perfusion supplies oxygen, substrates and regulatory agents to the tissues and washes out unnecessary compounds and waste. In addition to the situation with overt lack of perfusion leading to ischemia (e.g. due to vascular disease), perfusion has been found to be abnormal in many inflammatory and metabolic disorders. A number of efforts have been made to quantitatively, and non-invasively assess the tissue perfusion in vivo, in both preclinical and clinical experiments. Of those, PET provides unique characteristics, allowing 3-dimensional dynamic acquisition with very high sensitivity and accuracy. The methodology is based on kinetic modeling of the tracer behavior in the body, for several short-lived tracers such as 82Rb, 13NH3, H215O etc. This talk provides an overview of theory and application of the technique that quantitatively assess regional myocardial blood flow using PET in clinical and preclinical settings. Following issues will be discussed:
- Basic principles for quantitative assessment of regional myocardial blood flow from PET images.Compartmental model approachesWhat to assume, and how to model?
- Characteristics and kinetic modeling for typical radio-labeled tracers: 82Rb, 13NH3, H215O for PET, and 99mTc-ligands and 201Tl for SPECT, etc.
- Intrinsic limitations and error factors which need to be taken into account. Partial volume effectFirst-pass extraction fraction of the radio tracerRetention of the tracer in the myocardial tissueMetabolite production in the arterial bloodEstimation of the arterial input function from PET images
- Practical procedures, including the radiotracer production, PET scanning protocols, data analysis, etc.
- The procedures to generate quantitative images in PET, particularly when acquired in 3D mode. What corrections are needed, and how accurate are they?
- Example applications of quantitative assessment in ischemic cardiac diseases etc.
- Application of the technique to other organ tissue flow assessment
Relevant Publications:
- Koshino K, Watabe H, Enmi J, Hirano Y, Zeniya T, Hasegawa S, Hayashi T, Miyagawa S, Sawa Y, Hatazawa J, Iida H. Effects of patient movement on measurements of myocardial blood flow and viability in resting 15O-water PET studies. J Nucl Cardiol. 19(3);524-533,2012
- Hirano Y, Koshino K, Watabe H, Fukushima K, Iida H. Monte Carlo estimation of scatter effects on quantitative myocardial blood flow and perfusable tissue fraction using 3D-PET and 15O-water. Phys Med Biol. 57(22);7481-7492,2012
- de Haan S, Harms HJ, Lubberink M, Allaart CP, Danad I, Chen WJ, Diamant M, van Rossum AC, Iida H, Lammertsma AA, Knaapen P. Parametric imaging of myocardial viability using 15O-labelled water and PET/CT: comparison with late gadolinium-enhanced CMR. Eur J Nucl Med Mol Imaging. 39(8);1240-1245,2012
- Teramoto N, Koshino K, Yokoyama I, Miyagawa S, Zeniya T, Hirano Y, Fukuda H, Enmi J, Sawa Y, Knuuti J, Iida H. Experimental Pig Model of Old Myocardial Infarction with Long Survival Leading to Chronic Left Ventricular Dysfunction and Remodeling as Evaluated by PET. J Nucl Med. 52(5);761-768,2011
- Koshino K, Watabe H, Hasegawa S, Hayashi T, Hatazawa J, Iida H. Development of motion correction technique for cardiac 15O-water PET study using an optical motion tracking system. Ann Nucl Med. 24(1);1-11,2010
- Temma T, Iida H, Hayashi T, Teramoto N, Ohta Y, Kudomi N, Watabe H, Saji H, Magata Y. Quantification of Regional Myocardial Oxygen Metabolism in Normal Pigs using Positron Emission Tomography with Injectable 15O-O2. Eur J Nucl Med Mol Imaging. 37(2);377-385,2010
- Kudomi N, Koivuviita N, Liukko KE, Oikonen VJ, Tolvanen T, Iida H, Tertti R, Metsarinne K, Iozzo P, Nuutila P. Parametric renal blood flow imaging using [15O]H2O and PET. Eur J Nucl Med Mol Imaging. 36(4);683-691,2009
- Kudomi N, Slimani L, Jarvisalo M, Kiss J, Lautamaki R, Naum G, Savunen T, Knuuti J, Iida H, Nuutila P, Iozzo P. Non-invasive estimation of hepatic blood perfusion from H215O PET images using tissue-derived arterial and portal input functions. Eur J Nucl Med Mol Imaging. 35(10);1899-1911,2008
- Yokoyama I, Inoue Y, Kinoshita T, Itoh H, Kanno I, Iida H. Heart and Brain Circulation and CO2 in Healthy Men. Acta Physiol (Oxf). 193(3);303-308,2008
- Iida H, Eberl S, Kim KM, Tamura Y, Ono Y, Nakazawa M, Sohlberg A, Zeniya T, Hayashi T, Watabe H. Absolute quantitation of myocardial blood flow with 201Tl and dynamic SPECT in canine: optimisation and validation of kinetic modelling. Eur J Nucl Med Mol Imaging. 35(5);896-905,2008
- Watabe H, Jino H, Kawachi N, Teramoto N, Hayashi T, Ohta Y, Iida H. Parametric imaging of myocardial blood flow with 15O-water and PET using the basis function method. J Nucl Med. 46(7);1219-1224,2005
- Hove JD, Iida H, Kofoed KF, Freiberg J, Holm S, Kelbaek H. Left atrial versus left ventricular input function for quantification of the myocardial blood flow with nitrogen-13 ammonia and positron emission tomography. Eur J Nucl Med Mol Imaging. 31(1);71-76,2004
- Tadamura E, Mamede M, Kubo S, Toyoda H, Yamamuro M, Iida H, Tamaki N, Nishimura K, Komeda M, Konishi J. The effect of nitroglycerin on myocardial blood flow in various segments characterized by rest-redistribution thallium SPECT. J Nucl Med. 44(5);745-751,2003
- Iida H, Hayashi T, Eberl S, Saji H. Quantification in SPECT cardiac imaging. J Nucl Med. 44(1);40-42,2003
- Tadamura E, Iida H, Matsumoto K, Mamede M, Kubo S, Toyoda H, Shiozaki T, Mukai T, Magata Y, Konishi J. Comparison of myocardial blood flow during dobutamine-atropine infusion with that after dipyridamole administration in normal men. J Am Coll Cardiol. 37(1);130-136,2001
- Yamamoto Y, de Silva R, Rhodes CG, Araujo LI, Iida H, Rechavia E, Nihoyannopoulos P, Hackett D, Galassi AR, Taylor CJ, et al. A new strategy for the assessment of viable myocardium and regional myocardial blood flow using 15O-water and dynamic positron emission tomography. Circulation. 86(1);167-178,1992
- Iida H, Kanno I, Takahashi A, Miura S, Murakami M, Takahashi K, Ono Y, Shishido F, Inugami A, Tomura N, et al. Measurement of absolute myocardial blood flow with H215O and dynamic positron-emission tomography. Strategy for quantification in relation to the partial-volume effect. Circulation. 78(1);104-115,1988
Acknowledgements: Strategic Japanese-Finnish Research Cooperative Program entitled “Novel system for multi-organ quantitative tissue perfusion” supported by Japan Science and Technology Agency (JST) and Academy of Finland (AF)
Presentation 4: Modelling Applications in Oncology Studies
Adriaan A. Lammertsma
Radiology & Nuclear Medicine, VU University Medical Center, Amsterdam, Netherlands
Learning Objectives:
- Understand the need for quantification in response monitoring
- Understand the difference between semi-quantitative and fully quantitative approaches
- Obtain an overview of modelling in oncological studies
The two most important characteristics of PET are its quantitative nature and its high sensitivity. In oncology, its sensitivity has been used successfully, in particular for detecting (distant) metastases using [18F]FDG. . This has led to a significant improvement in staging with direct consequences for selecting the most appropriate therapy. It is clear that, for detecting metastases, quantification is not needed, as one only needs to find out whether they are present.
Based on its success in staging, PET increasingly is used to monitor response to therapy. In fact, [18F]FDG PET already is used as a surrogate endpoint in the development of new anticancer drugs. Clearly, visual assessment of response scans has limited value, as it can only be used in extreme cases. Quantification is needed for evaluating more subtle changes. In addition, it is needed to define objective cut-off points for multi-centre trials. Finally, visual assessment makes the inherent assumption that normal tissue (i.e. background) is unaffected by the therapy. This assumption may not be correct and is not needed in quantitative assessments.
The majority of [18F]FDG PET response studies have been analysed using the Standardised Uptake Value (SUV), which represent uptake in a tumour normalised to injected dose and body weight (normalisations to body surface area and lean body mass have also been used). The advantage of SUV is that it combines a static (whole body) scanning protocol with a simple way of analysing the data. It is, however, sensitive to various patient and protocol related factors. Consequently, significant efforts have gone into harmonizing acquired data with guidelines formulated by, for example, EORTC, NCI and EANM.
There is no doubt that, for many therapies, measured [18F]FDG SUV changes provide valuable information on the response (early) during therapy. Nevertheless, SUV represents a measurement at a single time point (after injection) and it assumes that [18F]FDG availability is predictable, i.e. (after normalisation) is the same from patient to patient and from baseline to response scan. With novel therapies this is not necessarily the case. For example, if a therapy results in an inflammatory response in other parts of the body, there will be increased [18F]FDG uptake in those inflammatory tissues, leading to faster clearance from plasma. This, in turn, will lead to lower net delivery to the tumour and the relationship between SUV and the real parameter, glucose metabolism, will be change. Consequently, in those cases, misleading information will be obtained when comparing SUV before and after therapy, and proper results can only be obtained by full kinetic analyses of dynamic PET data.
Another application of tracer kinetic modelling in oncology is the prediction of response to anticancer therapy. In this case the anticancer drug itself is labelled and tracer amounts are injected prior to any therapy. Tumour rate constants are derived from such a tracer study and, combining those rate constants with the plasma profile during therapy, may provide a means to predict whether tumour concentrations of (cold) drug will be high enough for a therapeutic effect.