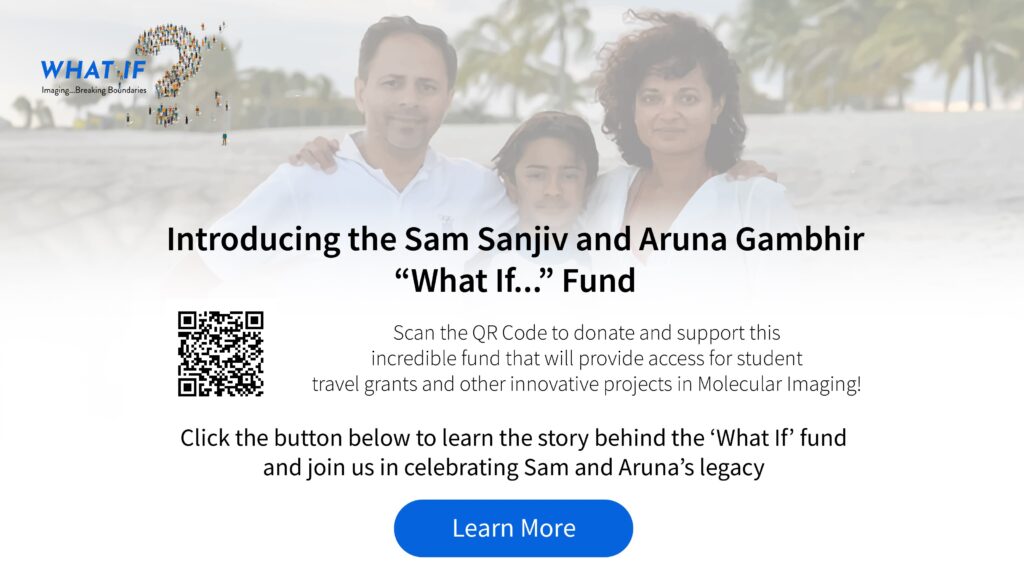
This emphasis covers computational and methodological approaches to molecular imaging data, including modeling, image analysis, image processing and quantification.
Machine Learning: Applications
Pre-clinical or clinical studies that apply machine learning methods to analyze molecular imaging data form the basis of this category. The focus is on the use or evaluation of machine learning algorithms for applications in molecular imaging studies and analysis, as well as the inclusion of big data in studies.
Machine Learning: Basic Developments
Basic developments include new algorithms or conceptual approaches for statistical analyses that are broadly applicable to imaging or other data. This includes methods to improve the efficiency of machine learning (transfer learning) and other investigations into the development of machine learning itself.
Modeling & Quantification
This category covers the use, evaluation and novel approaches for modeling, quantifying and analyzing imaging data. This includes kinetic modeling of PET or other dynamic datasets, biological or biochemical models and simulations. Any new analytical or numerical approaches to quantify imaging data is appropriate for this category.
Image Pre/Post-processing
Novel methods to process or analyze imaging data are considered in this category. This can include pre- or postprocessing methods that address motion, physiology or other noisy parameters. It also includes new approaches in image registration, especially for multi-modal imaging data, and methods involving image reconstruction. Any methods that improve image quality, sensitivity or accuracy fall into this category.